Ehrenberg-Bass researchers readily admit that consumers are heterogeneous in their brand preferences. Yet, they ignore the possibility that consumers also vary in their expected responsiveness to advertising.
If consumers are different in one way, shouldn’t they be different in the other?
Math says they must be. Heterogeneity of brand preference means heterogeneity of expected response to advertising in predictable ways. Here is what the curves look like. You see that the mode for probability of purchase occurs around 0 (most consumers have no interest in your brand) while that is also the lowest point of expected response to advertising. The circle highlights ad waste engineered by broad reach media plans.
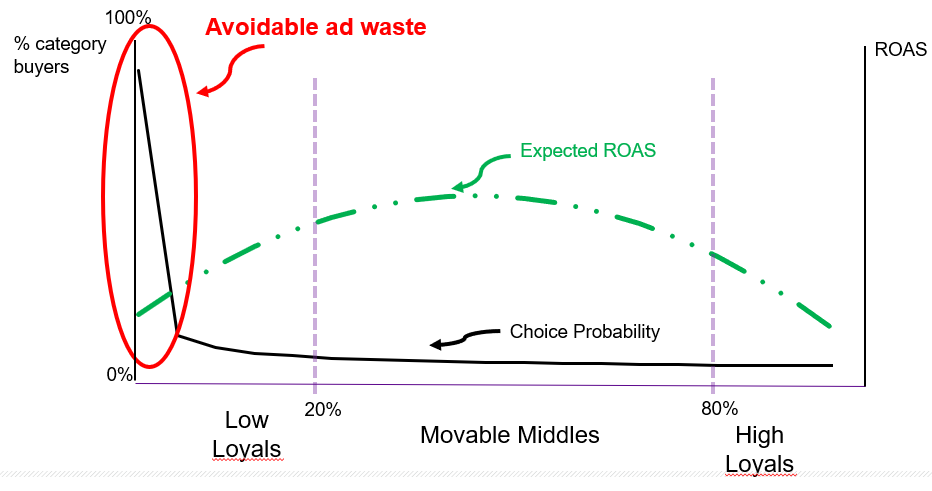
To see the connection, all an analyst needs to do is apply a little calculus to the logit function used in most MTA studies. They would derive that a category buyer’s expected conversion response to a given brand’s advertising is proportional to a simple mathematical expression p*(1-p) (where p is the baseline probability of purchase for a given consumer.) (The first derivative and expected ROAS are actually the same thing.) If everyone’s “p” is different, so is their p*(1-p).
This simple expression could change marketing practice.
First, it is the reason that broad reach can never be an optimal ad strategy despite EBI’s and Les Binet’s support for the idea “buy as much reach as you can afford”. Broad reach would work best only if the “Bayesian prior” expectation of consumer response was uniform across all consumers. But it isn’t; it is proportional to p*(1-p).
This simple expression is why we expect Movable Middles to be 5+ times more responsive to advertising than low loyals (mostly non-buyers), now supported in 10 out of 10 advertising responsiveness experiments. In fact, we see Movable Middles being up to 23 times more responsive to advertising vs. low loyals based on case study evidence.
This simple expression tells us how to win new buyers too.
Take two consumers…Consumer A who has a 1% probability of choosing your brand and Consumer B who has a 5% likelihood. Both are low loyals and almost certainly non-buyers. 1% vs. 5% doesn’t sound like a lot but our simple expression tells us that consumer B is expected to be 4.8 times more winnable.
Targeting more winnable non-buyers. Different addressable audiences will have different mixes of winnable vs. non-winnable non-buyers. The idea is simple…target disproportionately more media to audiences with a higher percentage of winnable non-customers. Furthermore, the winnability of non-buyers in a given audience is easy to estimate…all you really need is an estimate of the market share you have within each given audience which can vary 5:1 or more from highest to lowest share. This lets you fit a Beta distribution to each audience and that gives you the average winnability of non-buyers in each respective audience. Then fish where the fish are.
Ehrenberg-Bass could do this with their Dirichlet model (basically a Beta distribution fit for all brands simultaneously) and I would encourage them to do so.
Over the past six months, working with the MMA, I have taken Movable Middle theory and our simple expression a step further into creating an audience optimization tool. I have tested broad reach media plans for optimality in terms of either driving ROAS or new buyers. I have modeled brands with $100MM ad budgets, up to $2 Billion ad budgets. I have yet to find a scenario where broad reach is the best media allocation strategy.
The MMA is very interested in working with marketers to conduct a series of bake-offs among their actual media allocations, broad reach, and optimal solutions using the Audience Optimizer. This is a 3 week exercise at little or no cost and all you have to do is contact me to get the ball rolling (joel@rubinsonpartners.com).